A core tenet of the emerging field of radiomics is that modern, high-resolution CT imaging contains information that is invisible to the human eye, but that can be extracted and analyzed using image processing and computer vision techniques. In this project we are applying this insight to CT images of patients with metastatic liver tumors resulting from colorectal cancer.
Colorectal cancer is the second leading cause of cancer-related mortality in the United States. More than 50% of patients with colorectal cancer will develop liver metastases in their lifetime with a dismal <10% surviving past three years. Our goal is to leverage all information contained in CT imaging of patients with colorectal liver metastases (CRLM) to better understand the disease course and treatment response as a necessary step toward improving patient outcomes.
Our large interdisciplinary team of experts, combined with the largest clinical experience in CRLM in the western world makes this project a unique and unrivaled opportunity to define radiomics of CRLM. In the future, integration into existing clinical workflows means that small medical centers without highly specialized radiology groups would benefit from predictive algorithms developed at two high-volume centers via a low-cost software update. Successful completion of our aims will provide validated prognostic imaging markers with a pathway to routine clinical use, which are of paramount importance to improving patient survival of this deadly disease.
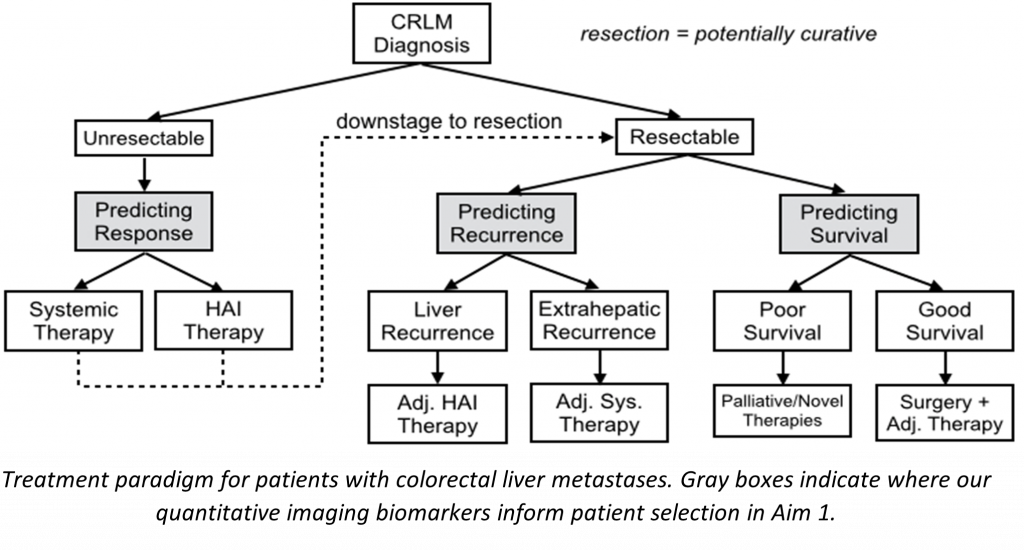
Project Aims
The objectives of this project are to develop and validate robust imaging features by standardizing image acquisition, to improve automated tools for clinical trial use, and to validate the predictive power of imaging features with external data. To pursue these goals, we have assembled an interdisciplinary team of experts in surgery, medical oncology, pathology, radiology, biostatistics, and image analysis, from Memorial Sloan Kettering Cancer Center (MSK), University of Texas MD Anderson Cancer Center (MDA), Rensselaer Polytechnic Institute, GE Research, and Queen’s University.
The project can be broken down into three aims:
- Development and validation of imaging features and models that are predictive of treatment response and recurrence of CRLM when undergoing chemotherapy or hepatic resection. These models will be developed and validated using 2450 retrospectively acquired CT images from both MSK and MDA, the two largest liver cancer centers in the United States.
- Systematic analysis of the repeatability and reproducibility of radiomic imaging features across CT imaging protocols through the prospective collection of test-retest imaging data. CT images are being collected at multiple time points and reconstructed with a variety of parameters in order to determine which radiomic features are robust to variations in CT acquisition protocol, and thus suitable for use across centers.
- Recapturing even more data from CRLM imaging by developing “rawdiomics” models that fully utilize CT sinogram data. Reconstruction algorithms that transform the raw sinogram data collected by scanners into human-readable images lose information in the process. By operating on the raw data, rawdiomics models will be able to better leverage the complete data collected by CT scanners. Raw sinogram data is being prospectively collected at both MSK and Queen’s University.
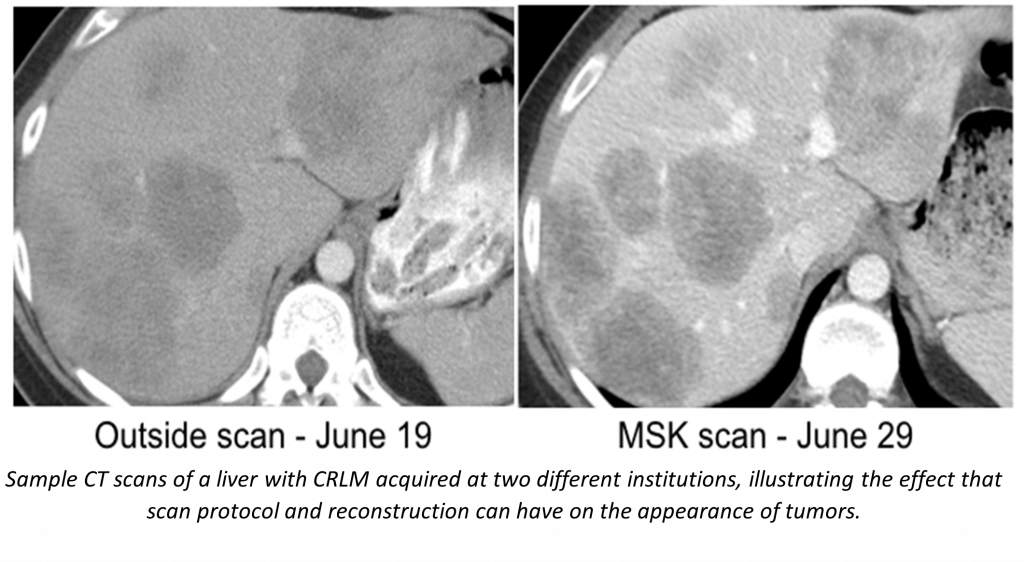
In support of these studies we are developing a number of software tools including an automated segmentation pipeline using state of the art machine learning, a tool for remote viewing and editing of model-produced segmentations, and a radiomic feature extraction pipeline.
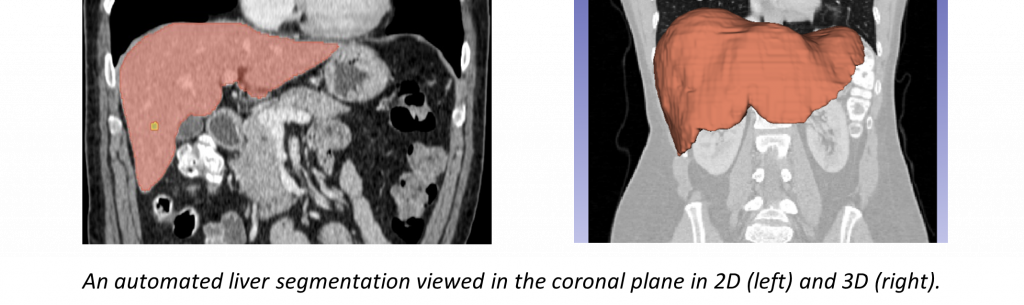
Publications
- Fan F, Xiong J, Wang G. Universal approximation with quadratic deep networks. Neural networks: the official journal of the International Neural Network Society. 2020 April;124:383-392. PubMed PMID: 32062373; PubMed Central PMCID: PMC7076904; DOI: 10.1016/j.neunet.2020.01.007.
- Fan F, Xiong J, Wang G. Universal approximation with quadratic deep networks. Neural networks: the official journal of the International Neural Network Society. 2020 April;124:383-392. PubMed PMID: 32062373; PubMed Central PMCID: PMC7076904; DOI: 10.1016/j.neunet.2020.01.007.
- Fan F, Xiong J, Wang G. Universal approximation with quadratic deep networks. Neural networks: the official journal of the International Neural Network Society. 2020 April;124:383-392. PubMed PMID: 32062373; PubMed Central PMCID: PMC7076904; DOI: 10.1016/j.neunet.2020.01.007.
- Fan F, Shan H, Kalra MK, Singh R, Qian G, Getzin M, Teng Y, Hahn J, Wang G. Quadratic Autoencoder (Q-AE) for Low-Dose CT Denoising. IEEE transactions on medical imaging. 2020 June;39(6):2035-2050. PubMed PMID: 31902758; PubMed Central PMCID: PMC7376975; DOI: 10.1109/TMI.2019.2963248.
- Fan F, Shan H, Kalra MK, Singh R, Qian G, Getzin M, Teng Y, Hahn J, Wang G. Quadratic Autoencoder (Q-AE) for Low-Dose CT Denoising. IEEE transactions on medical imaging. 2020 June;39(6):2035-2050. PubMed PMID: 31902758; PubMed Central PMCID: PMC7376975; DOI: 10.1109/TMI.2019.2963248.
- Fan F, Shan H, Kalra MK, Singh R, Qian G, Getzin M, Teng Y, Hahn J, Wang G. Quadratic Autoencoder (Q-AE) for Low-Dose CT Denoising. IEEE transactions on medical imaging. 2020 June;39(6):2035-2050. PubMed PMID: 31902758; PubMed Central PMCID: PMC7376975; DOI: 10.1109/TMI.2019.2963248.
- Lyu Q, Shan H, Steber C, Helis C, Whitlow C, Chan M, Wang G. Multi-Contrast Super-Resolution MRI Through a Progressive Network. IEEE transactions on medical imaging. 2020 September;39(9):2738-2749. PubMed PMID: 32086201; PubMed Central PMCID: PMC7673259; DOI: 10.1109/TMI.2020.2974858.
- Creasy JM, Cunanan KM, Chakraborty J, McAuliffe JC, Chou J, Gonen M, Kingham VS, Weiser MR, Balachandran VP, Drebin JA, Kingham TP, Jarnagin WR, D’Angelica MI, Do RKG, Simpson AL. Differences in Liver Parenchyma are Measurable with CT Radiomics at Initial Colon Resection in Patients that Develop Hepatic Metastases from Stage II/III Colon Cancer. Annals of surgical oncology. 2021 April;28(4):1982-1989. PubMed PMID: 32954446; PubMed Central PMCID: PMC7940539; DOI: 10.1245/s10434-020-09134-w.
- Pulvirenti A, Yamashita R, Chakraborty J, Horvat N, Seier K, McIntyre CA, Lawrence SA, Midya A, Koszalka MA, Gonen M, Klimstra DS, Reidy DL, Allen PJ, Do RKG, Simpson AL. Quantitative Computed Tomography Image Analysis to Predict Pancreatic Neuroendocrine Tumor Grade. JCO clinical cancer informatics. 2021 June; 5:679-694. PubMed PMID: 34138636; DOI: 10.1200/CCI.20.00121.
Abstracts
- Raney F, Do RK, Simpson AL. Toward Generalizable Semantic Medical Image Segmentation. Proceedings of the 18th Annual Symposium Imaging Network Ontario. 18th Annual Symposium Imaging Network Ontario; 2020 March 26.
- Hamghalam, M., Frangi, A. F., Lei, B., & Simpson, A. L. (2021, September). Modality Completion via Gaussian Process Prior Variational Autoencoders for Multi-modal Glioma Segmentation. In International Conference on Medical Image Computing and Computer-Assisted Intervention (pp. 442-452). Springer, Cham.
People
Advisors
Contributors
Current
- Gangai, Natalie
- Hamghalam, Mohammad
- Lasso, Andras
- Peoples, Jacob
- Tran, Anh
- Ungi, Tamas
- Williams, Travis
Former
- Ayhan, Miranda
- Cong, Wenxiang
- Haneda, Eri
- James, Imani
- Kang, Hyuns
- Lorraine, Peter
- Lyu, Qing
- Meng, Bob
- Niu, Chuang
- O’Grady, Brandon Blake
- Raney, Fraser
- Shan, Hongming
- Zhang, Yaoting
Downloads
Code Repositories
DICOM Segmentation Conversion
Code to convert segmentation label maps with associated DICOM CT images into standard DICOM Segmentation objects is available on Github: https://github.com/lassoan/LabelmapToDICOMSeg
Shared Datasets
TCIA Collection: Colorectal Liver Metastases, MSKCC
We have collected preoperative hepatic CT scans, clinicopathologic data, and recurrence/survival data, from a large, single-institution series of patients (n=198) who underwent hepatic resection of CRLM. For each patient, we also created segmentations of the liver, vessels, tumors, and future liver remnant. The largest of its kind, this dataset is a resource that may aid in the development of quantitative imaging biomarkers, and machine learning models, for the prediction of post-resection hepatic recurrence of CRLM.
This data set is being shared, in standard DICOM format, through The Cancer Imaging Archive (TCIA) under collection name “Colorectal Liver Metastases, MSKCC”.
Supporting Grant: This work is partly funded through the NIH supported grant R01CA233888.